
Supercharge clinical analytics workflow with clinical reasoning AI agents.

As the product and design lead for Mendel AI's Hypercubes, I was tasked with creating a healthcare data platform that could serve diverse stakeholders while addressing complex, interconnected challenges across the healthcare ecosystem. This case study demonstrates how systems thinking guided our design process, enabling us to create a holistic solution that transforms fragmented healthcare data into actionable insights.
Systems Analysis: Understanding the Healthcare Ecosystem

I began by mapping the complex network of healthcare stakeholders and their interdependencies:
This revealed five key stakeholder groups with distinct but interconnected needs:
-
Academic Medical Centers
-
Healthcare Providers
-
Diagnostic Companies
-
Data Aggregators
-
Pharmaceutical Companies
Problem Space Mapping
Through analyzing 1,267 customer conversations, I identified 16 critical pain points across these stakeholders. Rather than viewing these as isolated problems, I created a system map showing how these challenges interconnect and cascade:
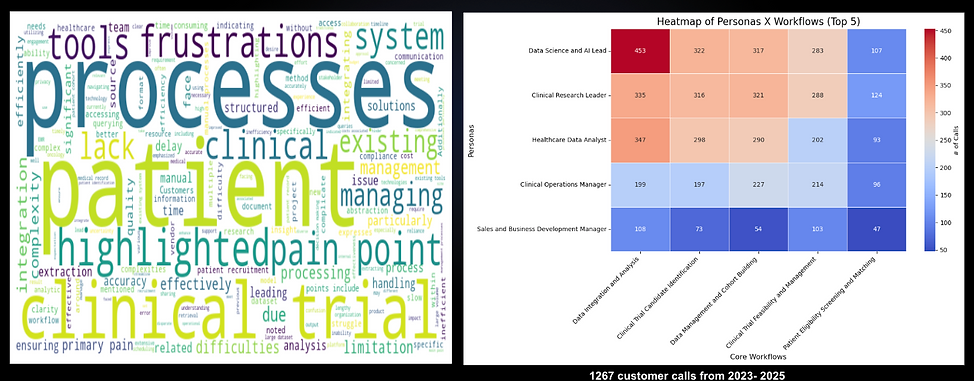
This revealed four fundamental root challenges that propagate throughout the healthcare ecosystem:
-
Data fragmentation across systems and modalities
-
Disconnection between clinical data and actionable insights
-
Manual workflows creating bottlenecks in data utilization
-
Siloed approaches to data analysis preventing holistic patient understanding
Design process & Decision making
We explored multiple directions but focused on a modular, metadata-driven architecture because it would allow us to address interconnected challenges while providing flexibility for different use cases. I led the development of a unified design system that could maintain context as users moved between different modules while accommodating the unique mental models of various healthcare roles.
For example, I created a cohort builder that serves as both a powerful research tool and a clinical decision support system:
-
For researchers, I designed advanced temporal filtering and comparison tools
-
For clinicians, I emphasized guideline adherence visualization and next-best-action recommendations
-
For data analysts, I prioritized cost and utilization pattern identification
We tested these concepts with users from each stakeholder group and discovered that maintaining consistent information architecture while adapting visualization styles to each context was critical. Based on this feedback, we iterated toward a declarative app model that could flexibly address different needs while maintaining system integrity.
A key turning point came when we realized that traditional search paradigms were insufficient for healthcare discovery. I prototyped an approach that combined conversational AI, chart visualizations, and literature insights into a unified discovery interface. Users responded enthusiastically to this integrated approach, which led us to develop the "Discovery Beyond Search" concept as a core platform theme.
Design framework - platform architecture principles
Unified data foundation - Creating connections across previously siloed data sources

Contextual Intelligence Layer - Transforming raw data into meaningful healthcare contexts

Workflow Integration Framework - Embedding insights within existing user workflows

Adaptive value generation - Enabling the platform to deliver different value for different stakeholders
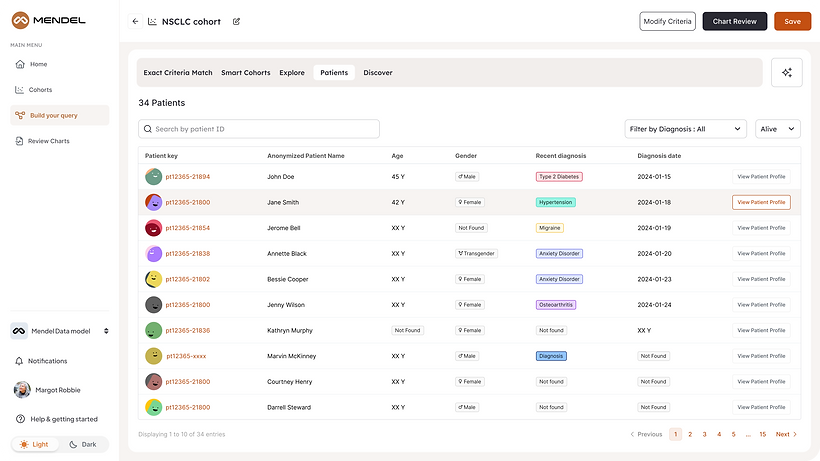

Outcome & Impact
The final solution was a comprehensive healthcare data platform with four core themes: declarative app architecture, expanded deployment models, hypergraph-based structured data support, and discovery beyond search. After initial deployment with key customers, we saw:
60% reduction in chart review time for clinical research coordinators
35% increase in guideline adherence tracking accuracy for providers
40% improvement in patient-to-trial matching efficiency
Customer feedback validated our systems approach, with one pharmaceutical partner noting: "Hypercube doesn't just solve one problem—it transforms how we connect clinical data to research outcomes across our entire organization."